Is your connected car smart enough
Intelligent mobility is a major challenge that the automobile industry is facing as the digital landscape transforms. With the evolution of information and communication technologies and cooperative intelligent transport systems (C-ITS), car manufacturers can now embed mobility devices in almost every automobile. However, these new systems and services, such as emergency brake assistance or connected traffic light controllers, involve new risks and larger attack surfaces.
How AI can boost digital trust in autonomous cars
The Trusted Autonomous Mobility (TAM) project launched last January helps car manufacturers and autonomous shuttle providers overcome the critical cybersecurity threats they face today. Our mission focuses on the security and reliability of the data exchanged by a vehicle with its direct environment, including other vehicles and the road infrastructure.
A new digital identity management solution called PKI was developed for C-ITS. It establishes a digital trust network within C-ITS for authorizing and securing the data shared by a vehicle in the ecosystem. To reinforce this digital trust, a complementary solution called misbehavior detection was designed to identify misbehavior from vehicles or road infrastructure with valid digital identities. At any time, the C-ITS network must protect itself from possible external and internal attacks.
An autonomous car driving in the city center will communicate with its ecosystem by exchanging secure messages. Its sensors detect the presence of objects or people, and the data is aggregated with other sources of information in the vicinity to ensure the integrity and authenticity of the exchanged messages. The data will then be analyzed and validated so the vehicle can rely on it to make decisions. To achieve this, the vehicle should perform plausibility and consistency checks on the messages received. If the message is suspicious, it will send a misbehavior report via a trusted road side unit (RSU) or by direct cellular communication (eNodeB). During the local detection, the vehicle may rely on AI to reject messages from the suspicious vehicle. A central entity called the misbehavior authority (MA) receives these misbehavior reports (MRs) and classifies the reported entities as malicious, faulty or guenine. In the testing phase, the MA intentionally transmits false data to test the sensor’s reliability, which helps create robust protocols that prepare the system to detect and prevent cyberattacks.
The MA is part of a global misbehavior detection solution based on AI, which consists of the following steps:
1. Collection
The MRs are stored in a NoSQL Database, adapted to the C-ITS environment where flexibility, scalability and efficiency are required.
2. Pre-processing
A first selection is performed by verifying the content of MRs received in order to remove incorrect MRs (incomplete MR, cryptographic consistency, data semantics, etc.) before going into the next step. Then, filters (spatial, temporal, etc.) are applied to group MRs by similarity.
3. Feature engineering
The pertinent kinematic data from the MRs (speed, position, acceleration) are extracted. New data such as the frequency of evidence received, and the difference between the kinematic data of two messages are created. The creation of these new data from the raw data of the MRs improves forecast accuracy.
4. Training and testing
This step consists of using supervised machine learning and neural networks (LSTM, GRU, MLP) to capture both the temporality and the statistics of the data to predict the type of misbehavior. During the training phase, we use labeled data to incrementally improve the model’s ability to predict whether a misbehavior is genuine. During this phase, we use performance metrics to classify problems and validate the model for its accuracy and determine the detection rate of the system. Upon completing the evaluation, the model goes into the testing phase, which helps decide its efficiency and performance in the actual environment and prepare the system to detect and prevent cyberattacks.
5. Response
The final objective is to identify if the vehicle is misbehaving and take appropriate actions. The MA can collaborate with manufacturers, OEM or law enforcement authorities to ensure misbehaving vehicles are disposed of appropriately. Depending on the type of misbehavior, the action may range from sending a simple notification to the driver to removing the vehicle from the trusted environment.
Implementation of this misbehavior detection process requires a substantial amount of upstream work on the data, an essential commodity. The datasets used to train the model are based on simulated data. The field experiments planned in the TAM project will support the validation of selected models with actual data.
The automotive industry is still in the early stages of integrating AI into its mobility solutions. However, as the connected vehicle ecosystem matures, we anticipate AI to play a more significant role in detecting and preventing cyberattacks. As a result, concepts that sound futuristic today, like detection and classification of objects through computer vision, will become the norm. These concepts and technologies will make it possible to solve many cyber challenges related to vehicle automation. Ultimately, mastering AI and integrating it into cybersecurity solutions will go a long way to ensuring the safety of users.
About the authors
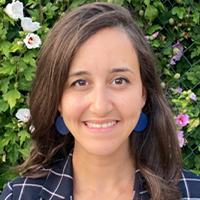
Hafeda Bakhti
Digital ID Innovation Team Leader, IDnomic, Atos
Graduate of Polytech Paris Sud and holder of an MBA at IAE Paris Sorbonne Business School, Hafeda is currently performing the role of innovation team leader at Atos IDnomic, with 7 years ’experience as a R&D engineer in Paris (France). She mainly works on ITS projects and participates in ITS research projects in partnership with IRT SystemX (French Research Institute). She also was involved in ITS deployment projects like the national French pilot deployment SCOOP@F, InterCor and C-Roads. She has managed the deployment of the Atos IDnomic ITS PKI solution in SaaS environment and led the product development in accordance with European regulations (C-ITS platform), ETSI standards or specific functionalities. Hafeda contributes in ETSI standardization efforts. She now focuses on the misbehavior detection in C-ITS with the aim of improving safety.
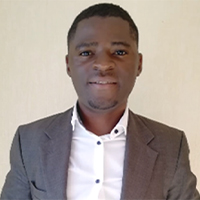
Guy Anthony Nama Nyam
Engineer R&D Innovation for Digital ID, Atos
Guy Anthony is a Data Scientist specialized in machine learning algorithms on large, complex, structured or unstructured data with several years of experience as a software engineer. As an engineer R&D Innovation with Atos, Anthony is mainly involved in ITS research projects in partnership with IRT SystemX (French Research Institute) and he contributes to ETSI standardization work. In addition to his job functions, he accompanies young people wishing to evolve in the field data.
Interested in next publications?
Register to our newsletter and receive a notification when there are new articles.